Introduction: A Paradigm Shift in the Payments Landscape
The payments industry is entering an era where intelligence is embedded into the very core of every transaction, terminal, and API. AI, Machine Learning (ML), and Generative AI (GenAI) are not just add-ons or efficiency boosters; they are becoming foundational layers of payment infrastructure, shaping everything from fraud prevention to merchant onboarding, from FX optimization to regulatory compliance.
As a product leader in this space, Iโve had the opportunity to build, evaluate, and scale payment platforms across APAC and the shift is unmistakable: payment providers who fail to adopt an AI-first mindset will not just lag behind, they will become obsolete.
This mega blog is broken down into three major sections:
- AI/ML in Product Infrastructure and Risk Management
- GenAI in Compliance, Treasury, and Monetization
- AI in Merchant Acquiring, POS, and O2O Experiences
Letโs deep dive into how AI is transforming the full payments value chain.
๐ A Quick Market Snapshot
- The global AI in fintech market is projected to grow from USD 42.83 billion in 2023 to USD 49.43 billion in 2024 at a CAGR of 15.4%.
- By 2030, it’s expected to reach USD 89.85 billion, demonstrating its rapid maturity in financial ecosystems.
This growth is not driven by hypeโbut by proven applications in:
- Enhanced fraud detection
- Scalable real-time processing
- Embedded compliance
- Smarter underwriting and scoring
- Seamless user experience
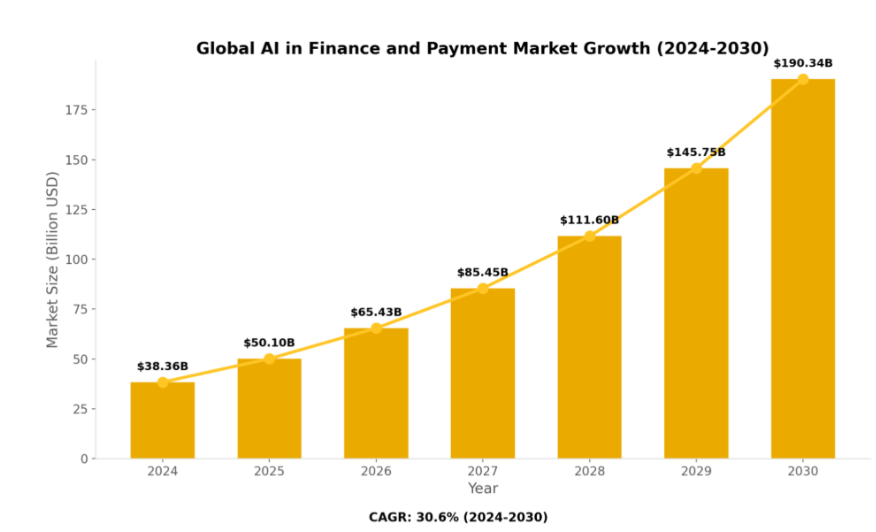
๐ The Transformative Role of AI in Payments
1. Scalability and Flexibility
AI enables payment systems to adapt dynamically to demand spikes. During events like Singles’ Day in China or Black Friday in the U.S., AI systems anticipate and scale capacity, preventing crashes and maintaining uptime.
2. Interoperability Across Ecosystems
AI-driven middleware and translation layers enable previously siloed systems to interact. Digital wallets, CBDC rails, POS systems, and even closed-loop cards can operate together through AI-driven APIs and orchestration engines.
3. Real-Time Processing
With ML algorithms continuously learning from transaction history and behavioral data, payments can now be processed and authenticated almost instantly, reducing wait times and minimizing false declines.
4. Security and Fraud Management
From device fingerprinting to AI-powered transaction pattern recognition, fraud prevention has become predictive rather than reactive. AI flags anomalies within milliseconds, reducing the window for fraudsters.
5. Superior Customer Experiences
AI powers chatbot assistants, voice-enabled interfaces, and contextual personalization. It can suggest credit top-ups, recommend EMI plans, or resolve disputes conversationally.
๐ง Core AI Technologies Shaping Payments
๐งฉ Machine Learning (ML)
ML models are used for:
- Behavioral fraud detection
- Merchant scoring
- Dynamic pricing & MDR optimization
- Customer segmentation
๐ฃ๏ธ Natural Language Processing (NLP)
NLP facilitates:
- Voice-based payments (e.g., “Pay electricity bill from UOB wallet”)
- Smart chatbots for support and onboarding
- Sentiment detection for customer satisfaction prediction
โจ Generative AI (GenAI)
GenAI is emerging in:
- Dynamic marketing campaigns
- Automated dispute resolution messaging
- KYC/KYB document interpretation
๐ Blockchain & Smart Contracts
When combined with AI:
- Blockchain supports transparent, traceable payments
- AI triggers conditional releases (escrow, invoice milestones)
๐ก๏ธ Biometrics & Computer Vision
AI enables accurate verification via:
- Facial and voice recognition
- Tamper-proof document scanning
- Liveness detection to counter spoofing attacks
๐งฎ Quantum AI (Emerging)
- Used in test cases to solve cryptographic challenges
- Accelerates large-scale fraud pattern detection
๐ RegTech
- Real-time AML scanning
- Automated regulatory interpretation (MAS, RBI, FATF)
๐ข Real-World Applications by Leading Companies
๐ PayPal โ AI for Fraud Detection
Processes billions of transactions using ML to detect velocity spikes, IP location mismatches, and device anomalies. Maintains fraud rates as low as 0.32%.
๐ก๏ธ Stripe โ Merchant Risk Scoring
Stripe’s Radar ML engine evaluates merchants at onboarding, assessing factors like site structure, SSL validity, and business model to auto-approve or escalate.
๐ Wise โ Cross-Border Payments
Optimizes corridor selection using AI for cost, speed, and compliance. Predicts FX trends and ensures FATF compliance in near real-time.
๐งพ Revolut โ Automated KYC
Uses AI to scan ID documents, run biometric checks, and trigger automatic verification. Reduces onboarding time to under 5 minutes.
โ๏ธ American Express โ IVR Intelligence
Enhances its phone-based payment systems with voice understanding, allowing users to interact via speech for bill payment and customer service.
๐ ๏ธ Implementing AI in Payment Infrastructure
To successfully embed AI:
- Assess existing tech stack and pain points
- Define use-case objectives (fraud, UX, onboarding)
- Choose tools (ML engines, GenAI models, RegTech suites)
- Ensure clean, labeled datasets
- Run pilots in sandboxed flows (e.g., AML or refund reconciliation)
- Integrate via secure APIs
- Train staff on AI behavior interpretation
- Iterate and scale by region, use case, or customer segment
โ ๏ธ Common Challenges (and Solutions)
Problem | AI-Powered Solution |
---|---|
Data privacy concerns | Implement federated learning & encryption |
AI bias | Use diverse training datasets, continuous validation |
Regulatory opacity | Deploy LLMs trained on local regulations |
Adversarial fraud | Behavior analytics + anomaly detection |
High cost | Start with modular tools, use open-source libraries |
๐ AI/ML & GenAI in Payment Product Infrastructure
๐ 1. AI-Powered Fraud Prevention
In the traditional world, rule-based engines were reactive: detect and block. But modern fraud patterns mutate faster than rules can be updated. AI flips this paradigm:
- Real-Time Behavioral Profiling: AI continuously learns individual user behavior patterns and flags deviations instantly.
- Graph AI: Models build connections across devices, users, cards, and IP addresses to detect fraud rings.
- Multi-Modal Signals: Combining device fingerprinting, geo velocity, typing speed, and merchant category into a unified trust score.
Use Case: A sudden change in time zone, device type, and transaction value for a user in Bangkok triggers a real-time challenge, saving thousands in potential chargebacks.
๐ค 2. AI in Risk-Based Pricing and Underwriting
Payment data is an under-leveraged asset in credit scoring and pricing. AI now allows:
- Dynamic Risk Pricing: Merchant MDRs and customer fees vary based on transaction health, dispute ratio, and location risk.
- Cashflow Lending: ML reads transactional cashflows to offer working capital in under 2 minutes.
- Underwriting-as-a-Service: Embedded credit lines for SaaS, ecommerce, and logistics partners based on payment trends.
๐ค 3. GenAI for Customer Interaction
Beyond chatbots, GenAI enables personalized, goal-driven interaction:
- Dispute Resolution Assistants: Drafts chargeback templates, retrieves past cases, and suggests evidence.
- Conversational Onboarding: A multilingual assistant for SME onboarding, especially in Vietnam, Indonesia, and India.
- Contextual Messaging: Dynamic content for payment notifications, KYB rejections, or promotional offers.
๐ 4. AI in Cross-Border Routing & FX
Cross-border B2C payments require sophisticated orchestration:
- AI FX Prediction: Predicts near-term exchange rate movements and advises settlement timing.
- Routing Optimizer: ML chooses best rail (Visa, UnionPay, local rails) based on latency, success rate, and cost.
- AI Swap Execution: Treasury bots execute FX swaps and pre-fund corridors based on forecasted flows.
๐ AI & GenAI in Compliance, Treasury & Monetization
โจ๏ธ 1. GenAI in Regulatory Compliance
Staying compliant across MAS, RBI, AUSTRAC, etc., is a full-time job. GenAI reduces the burden:
- Rule Interpretation Engines: AI parses PDF circulars, extracts obligations, and maps to existing policies.
- Audit Automation: Prepares compliance checklists, logs control implementation, and alerts for gaps.
- Real-Time Scanning: Detects upcoming regulatory updates and summarizes impacts for product, ops, and legal teams.
๐ 2. AI in AML & Sanctions Monitoring
AI brings radical accuracy to AML:
- Behavioral Clustering: Detects layered money movement across wallets, cards, and accounts.
- Adaptive Thresholds: ML adjusts velocity limits dynamically by geography and merchant type.
- Peer Analysis: Flags merchants whose refund ratios, transaction patterns deviate from peer cohorts.
๐ฐ 3. Treasury & Liquidity AI
Managing float and FX is key for PSPs:
- Intraday Liquidity Forecasting: AI predicts payout timelines, fund inflows, and wallet depletion.
- Idle Float Optimization: AI recommends float deployment across corridors to minimize FX risk.
- Automated Treasury Copilot: Executes FX at optimal spreads and simulates impact on P&L.
๐ 4. Commercial Strategy & GenAI in GTM
Beyond backend, AI is shaping front-office execution:
- Segment Intelligence: AI surfaces underserved but high-potential segments.
- Uplift Modeling: Predicts revenue lift from enabling new features per merchant category.
- GenAI for GTM: Creates personalized pitch decks, email campaigns, training content in seconds.
๐ 5. Smart APIs with Embedded AI
Modern APIs evolve with usage:
- Intent-Aware APIs: Detect misuse or inefficiency and self-correct (e.g., optimize retry logic).
- Merchant Support AI: A GenAI assistant reads logs, API keys, errors and suggests fixes.
- Dynamic SLAs: Predictive throttling and proactive rerouting based on traffic patterns.
๐จ AI/ML & GenAI in Merchant Acquiring & Physical Commerce
๐ 1. Intelligent Merchant Onboarding
Onboarding that adapts to risk, geography, and merchant profile:
- OCR + Entity Resolution: Extracts UBOs from documents and runs background checks.
- Dynamic KYB: SMEs get instant approval; high-risk merchants go through deeper vetting.
- Voice-Enabled Onboarding: Spoken document uploads and voice chat support in regional languages.
๐ข 2. AI at the Point-of-Sale (POS)
Terminals become growth engines:
- Real-Time Personalization: Contextual offers based on customer segment and past behavior.
- On-Device ML: POS systems work offline to flag double swipes, suspicious patterns.
- Inventory + Payments: Suggests product bundling based on historical combos.
๐ค 3. GenAI Merchant Copilot
Merchant service becomes 24×7 and intelligent:
- Weekly Pulse Reports: GenAI summarizes growth, refunds, product insights.
- Churn Prediction: Detects leading indicators of merchant drop-off.
- Autonomous Helpdesk: Answers questions like โWhere is my payout?โ or โWhy was this fee charged?โ
๐ 4. Offline-to-Online (O2O) AI Journeys
Bridge physical and digital journeys:
- Geo Fencing Offers: Reward repeat customers via mobile re-engagement.
- Smart QR Journeys: Tailored loyalty journeys and upsell at point of scan.
- WhatsApp Commerce: Post-purchase AI nudges that drive reorders.
๐ง 5. AI-First Merchant Platforms
Merchant portals now think:
- Auto UI Adaptation: UI adjusts based on merchant behavior.
- Reconciliation AI: Matches payments, fees, tax, and GL in seconds.
- Self-Driving Compliance: GST/VAT filings, threshold tracking, rule updates.
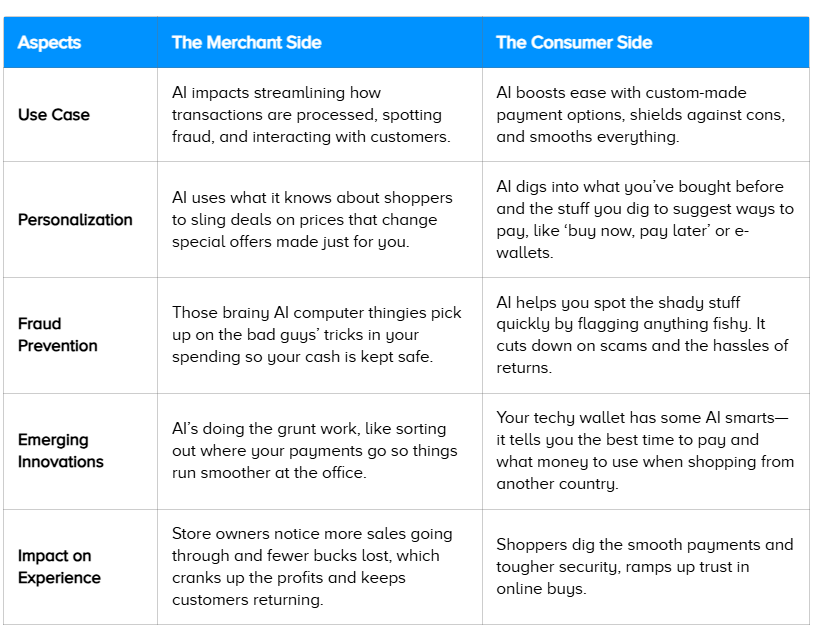
๐ Looking Ahead: Where AI in Payments is Going
Innovation Area | AI Use Case | Strategic Outcome |
---|---|---|
AI-Native PSPs | End-to-end AI product, support, ops | 10x leaner, faster payment firms |
Autonomous Onboarding | GenAI-led KYB & scoring | Reduce CAC by 70% |
Treasury AI | Intraday liquidity + FX intelligence | Optimize working capital & margins |
Conversational Payments | Voice, chat-based checkout | Better inclusion & UX |
Self-Healing APIs | Adaptive error-handling & throttling | Resilience and uptime |
The Road Ahead: Where Are We Headed?
Innovation Area | Near-Term (2025) | Mid-Term (2026โ27) | Long-Term (2028+) |
---|---|---|---|
GenAI Assistants | Dispute handling | End-to-end agent for RM & merchant ops | Autonomous product configuration |
AI Risk Scoring | Payment behavior tracking | Embedded credit lines | Real-time merchant-level dynamic pricing |
LLM Compliance | Regulatory summary generation | Rule impact simulation | Zero-touch compliance audits |
Future Use Cases: Whatโs Coming in 2026โ2028?
Theme | Use Case | GenAI Role |
---|---|---|
AI-Native PSPs | Launching PSPs with zero manual ops | GenAI as ops, support, compliance, marketing |
Compliance-as-a-Service | Plug-and-play AI modules for startups | Auto-generate AML policy, KYB journey |
AI-led Product Experimentation | Releasing and evaluating features in weeks | Simulate feature usage, write code, draft GTM |
What’s Next: Future of AI in Physical Commerce (2026+)
Innovation Area | AI/GenAI Use Case | Strategic Impact |
---|---|---|
Autonomous Merchant Acquisition | AI predicts which merchants to target next based on local footfall, transaction data, social signals | 10x sales productivity |
Voice-Enabled POS | Voice-based checkout, inventory checks, support | Multilingual market access |
Real-Time Tax & Compliance | AI pre-populates tax filings, reconciles GST/VAT | SME compliance without consultants |
GenAI Marketing Copilots | Merchants generate their own ads, promos, loyalty plans via GenAI | Zero human dependency for growth |
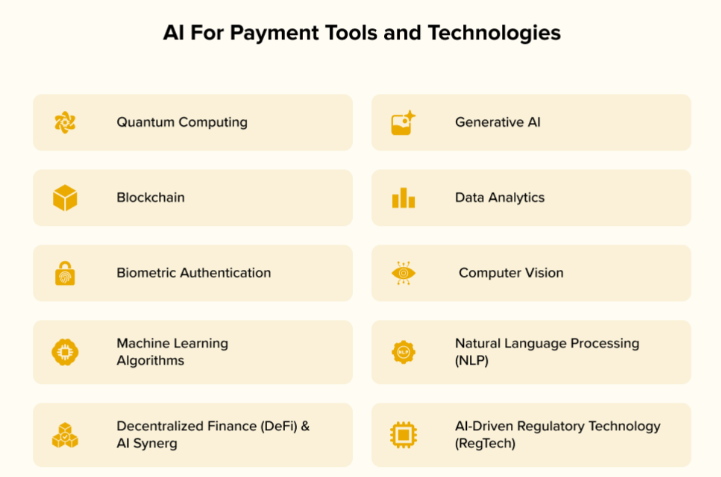
๐ Final Word: From Transactional to Intelligent
AI is not a feature. Itโs a foundational layer. It transforms every component of payments:
- Fraud prevention becomes prediction
- Onboarding becomes autonomous
- APIs become self-healing
- Compliance becomes real-time
- Treasury becomes a profit center
If you’re building for the future of payments, the question is no longer “Should we use AI?” but rather, *”Where can we embed AI so deeply that it becomes invisible?”
Letโs build platforms that think, adapt, and serve not just process.